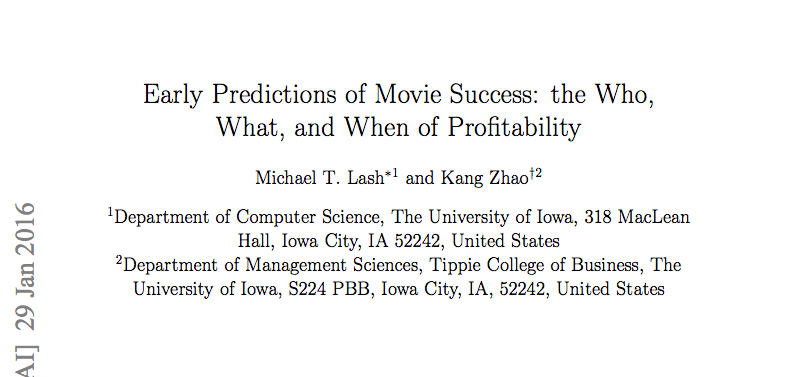
A new study from the University of Iowa confirms what has long been known in the industry – to predict the success or failure of a film is hard, but not impossible. The paper ‘Early Predictions of Movie Success: the Who, What, and When of Profitability‘ by doctoral student Michael T. Lash and business school professor Kang Zhao shows that there are some factors that can have more sway than others. For example, always be sure to hire Steven Spielberg and Tom Hanks.
Prof. Zhao and Mr Lash are said to have created an artificial intelligence (AI) algorithm that helps determine which films will turn a profit. The algorithm is based on a number of inputs, including cast members (“who”), the subject matter (“what”), and timing of the release (“when”), as well as a host of other factors. According to them:
Experiment results with movies during an 11-year period showed that the system outperforms benchmark methods by a large margin in predicting movie profitability. Novel features we proposed also made great contributions to the prediction.
As well as having practical implications on investment decisions, the paper could have implications for further theoretical research into how ‘team’ combinations of factors work and how success of creative works is quantified.
It is important to remember that how much money a film makes at the box office (and subsequent platforms) is only one factor that has to be weighed against the cost of production, as well as distribution and marketing. “Dead pool” has grossed USD $763 million world-wide, which is less than the global tally of USD $871 million for “Batman v Superman: Dawn of Justice”, but the former cost USD $58 million for the former while the latter officially cost $250 million (but possibly even as much as USD $410 million) to produce, meaning “Deadpool” earned more than 13 times its budget, while the DC adaptation ‘only’ doubled.
Of the 2,500+ films that the researchers included in their study, only around one-third were profitable, despite the fact that the researcher’s profitability threshold is lower than that of Hollywood studios, where famously even hits like “Coming to America” are judged to have made an accounting loss, in what is known as ‘Hollywood accounting‘.
The researchers used to measure of profitability: of a film earned more than USD $7.3 million above its budget or if it made at least an 11% return on investment. Given the revenue split between distributors and exhibitors, these measures only make sense if you consider then an indication of additional downstream revenue, which tends to be less early available and is hence calculated as a proportion of total box office earnings.
Yet what makes the research paper interesting is that it squarely puts itself in the position of a producer or investor, armed only with a limited set of information in the pre-production phase, such as the proposed cast, the essence of the script and the planned release. The research sees itself contributing in three areas in this early stage of movie development:
First, this decision support system is the first to harness machine learning, text mining, and social network analytics into one comprehensive decision-support system to predict movie profitability (rather than revenue), especially doing so during early stages of movie production, and with minimal human interventions.
Second, our research proposes several novel features, such as dynamic network features, plot topic distributions, the match between “what” and “who”, the match between “what” and “when”, and the use of profit-based star power measures. We showed that these features all contribute to the system’s performance in predicting profitability.
Third, our system is the first to collect different types of data (including structured data, network data, and unstructured data) from different freely-available sources, and fuse them for predicting the success of movies.
This is a serious and thoughtful approach to a complex challenge, even if it is not the first such attempt.
Previous Attempts to Predict Box Office Takings
There have been several previous efforts to predict the success of a movie, based on certain known factors:
There is Alec Kennedy’s paper “Predicting Box Office Success: Do Critical Reviews Really Matter?” from the University of Berkeley, which finds a correlation between positive reviews and a good BO performance, but significantly also “cannot find a significant amount of information to show that it is profitable to release a film in more theaters if they anticipate good reviews,” which means that distributors still don’t know where to push out a well-reviewed film to a larger number o screens.
Two years ago Google released a white paper by Andrea Chen called “Quantifying Movie Magic with Google Search.” This looked at the box office potential of a film based on the amount and type of online search that precedes a films release. Four weeks out from a given film’s release the amount of search volume for a trailer, combined with information such as franchise status and seasonality, the study claimed to predict opening weekend box office with an accuracy of 94% accuracy.
The limitation of the two able studies is that they only come into play when the film has already been completed and is ready to be reviewed and released. They would be of little help in terms of investment in a new film production.
Then there is ScriptBook, which is a software form a Belgian start-up that claims that it can predict how much money a script will make at the box office. ScriptBook was one of the ten startups that were highlighted at this year’s Berlinale’s European Film Market and in May the company raised €1.2 million seed round. But the software has not been demonstrated widely and even then does not take into consideration factors such as casting or release plans.
Stanford researcher Matt Vitelli’s “Predicting Box Office Revenue for Movies” from 2015 which sought to use the network representations of the Internet Movie Database (IMDB) “to build more powerful prediction models compared to common baseline methods.”
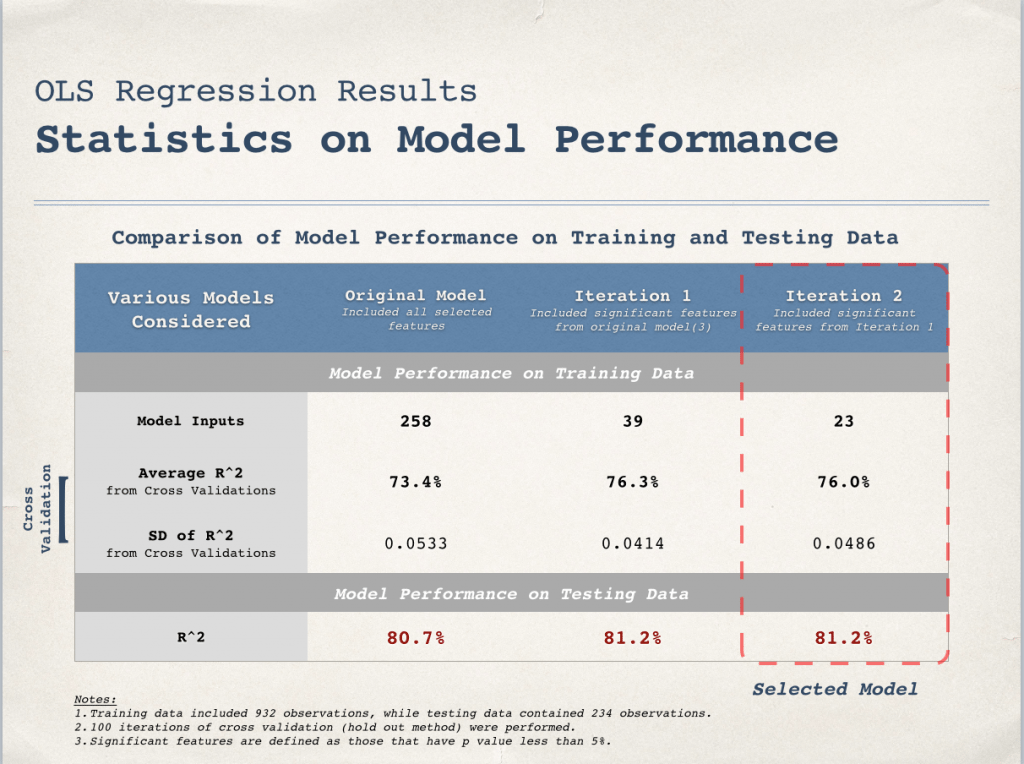
In a slightly humorous home-brew effort Adventures in Data Science’s Itelina set about last year to build a predictive model using five years of data scraped from BoxOfficeMojo and IMDB using linear regression. Would this work better than her husband’s gut feeling? Final result was 81% R Squared. How well did it do to predict top 20 box office hits? “I got 16 out of 20 correct! Dammit Jonny Depp for making your flops.”
Most closely linked to the latest paper is “Predicting Box-Offie Success of Movies in the U.S. Market” by Stanford’s Darin Im and Minh Thao Nguyen from 2011. It’s conclusion? “Although our model isn’t likely to be snatched up by movie executives anytime soon, it appears that predictive modeling of movie revenue in general is still a work in progress.” But the paper also effectively predicts Lash & Zhao’s paper:
However, we do believe that it would be possible to increase the accuracy of our model from its current state. Perhaps the most important change that we can make is to implement some feature to take into account the “star power” of a movie. Additionally we could take into account other features such as the origin country of the movie, writers, directors, and whether it was released on a holiday weekend.
This is precisely what is being attempted in the University of Iowa research, which taps into more powerful data sets and computing power than was possible even as recently as four years ago.
The Findings
In matching up determining factor, the research found that in pairing up the top 20 directors and male actors, Steven Spielberg and Tom Hanks came top, with hits such as “Catch Me If You Can”, “Saving Private Ryan” and “Bridge of Spies”. Samuel L. Jackson is the most ‘profitable’ actor on a stand-alone basis, not last by having been involved in both the Star Wars and Marvel franchises.
The algorithm also found that low-budget horrors were often a good return on investment, with films like “Paranormal Activity” costing little to make but earning USD $141 million in the US alone. An ‘R’ rating also shrinks the audience pool and makes return on investment more challenging. None of the above should come as a surprise to any seasoned industry watcher.
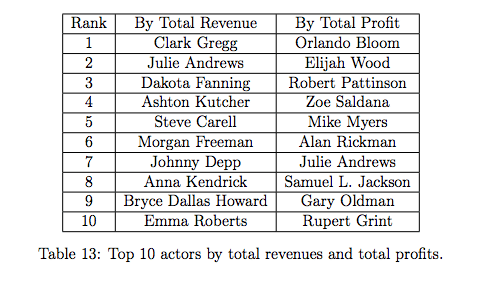
Where the algorithm throws up surprises is in showing that films with plot key words such as ‘war’, ‘mission’ and ‘fight’ tend to be unprofitable. The Drama Genre is only slightly less profitable than R-rated films. And films about music bands is not one you want to put your money into.
While the researchers themselves acknowledge the limitations of their prediction model, they see scope for improvements with data points gathered form more and deeper sources. Particularly for scripts.
Another interesting future direction for research would be to collect full-length scripts of a large number of movies and to then analyze the scripts, instead of the plot synopses. With movie scripts we can get more fine-grained topic distribution vectors, as well as many other novel features, such as script cadence. We also intend on adding more features to our model, including those that more definitively speak to consumer spending power, such as external economic indices, as well as those that take into account the types of movies that are most suited to certain times of the year (i.e. is it best to release Christmas-themed movies at Christmas time?).
If they succeed with this effort, then Christmas will have come early for film producers – most likely staring Samuel L. Jackson as Santa Clause.